Improving the Robustness of Object Detection Under Hazardous Conditions
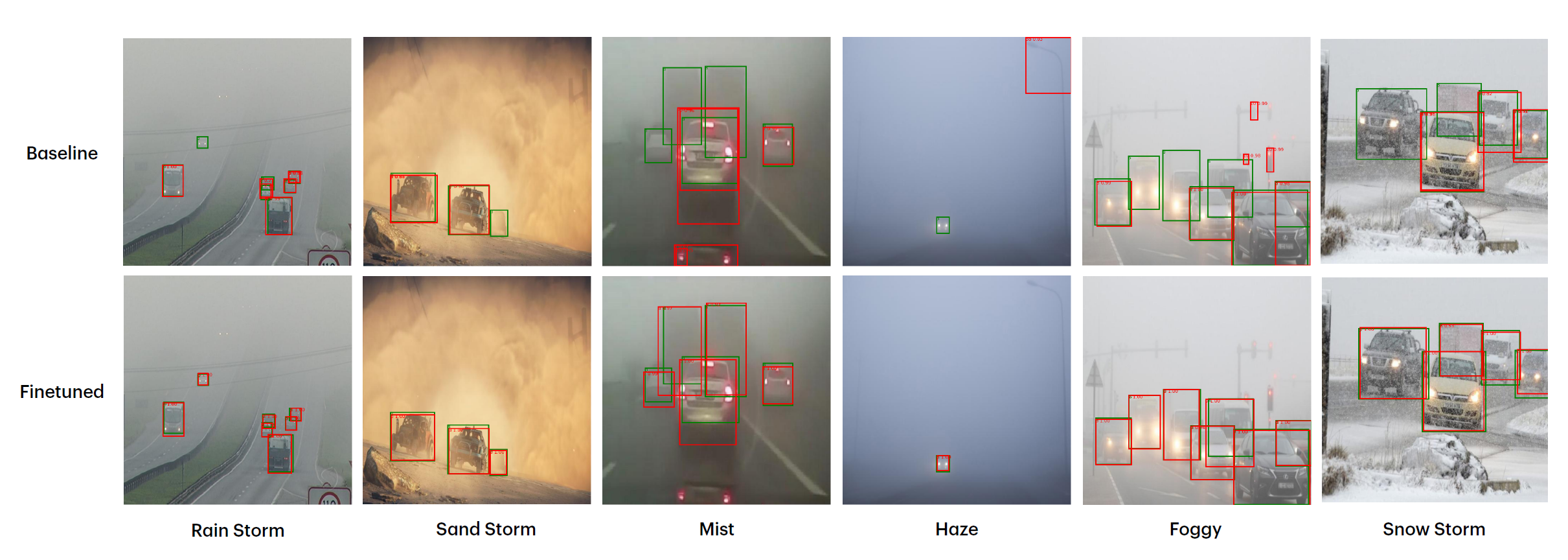
Abstract
In this project, we evaluated the baseline performance of DETR (Carion et al., 2020), a transformer-based object detector, under adverse weather conditions using the DAWN dataset. We improved detection robustness by fine-tuning both the CNN backbone and transformer decoder, allowing the model to better handle low-visibility scenarios like rain, snow, fog, and sandstorms. Data augmentation techniques were also applied to simulate hazardous conditions and enhance generalization. Our results show that fine-tuning on domain-specific datasets improves performance, though challenges remain when dealing with diverse and severe weather. Future work will focus on integrating de-weathering modules to further boost detection reliability.
Results
We compare the baseline model with two versions: a finetuned model (“Finetuned”) and a deweathered model (“De-weathered”). Among the eight weather types in the DAWN dataset (Kenk and Hassaballah, 2020), ‘dusttornado’ is excluded due to having only two duplicate samples. Results are reported for six weather types — rain storm, sand storm, haze, mist, foggy, and snow storm — with sample counts ranging from 7 to 28. Evaluation follows the baseline metrics, using six Average Precision (AP) and six Average Recall (AR) scores. AP and AR are further broken down by object size (small, medium, large) and by maximum detections allowed (1, 10, 100). The IoU=0.5:0.95 metric represents an average over multiple IoU thresholds. The details in the result comparison for each test case can be found in the report. We also used the finetuned model on a bad weather driving vedio as shown below.
Project Video
Contact
If you have any questions, feel free to contact Inbum Park.